Bioimage model zoo - a community-driven resource for accessible deep learning in bioimage analysis
Technologies: BioImage Model Zoo, CARE and ZeroCostDL4Mic
Preprint published in BioRxiv, January 2022
Publisher: Cold Spring Harbor Laboratory
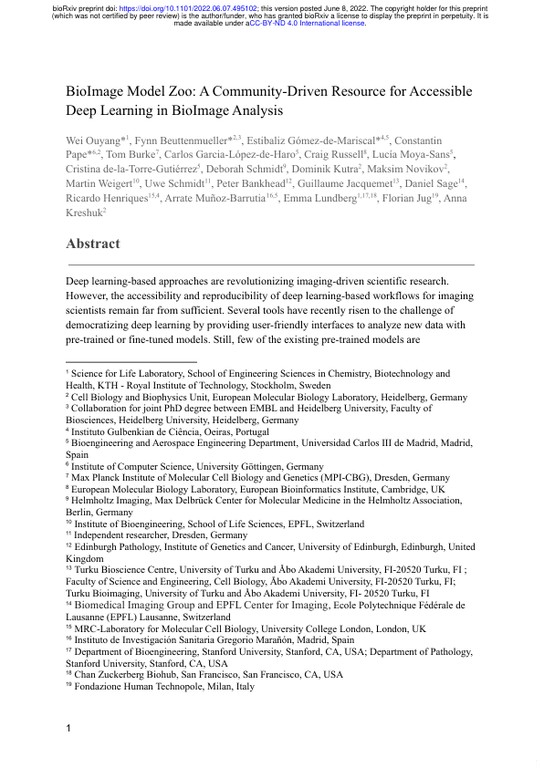
The manuscript introduces the BioImage Model Zoo, a community-driven resource for sharing, exploring, testing, and downloading standardized pre-trained models for deep learning (DL) in bioimage analysis. The Zoo aims to make DL methods for microscopy imaging findable, accessible, interoperable, and reusable (FAIR) across software tools and platforms. It supports a unified way of describing and consuming trained DL models through a standard model description format and libraries for standardized model execution. The Zoo is already supported by several tools, including ilastik, deepImageJ, ImJoy, StarDist, ZeroCostDL4Mic, and QuPath, and is open to new community partners. The manuscript also discusses the development and implementation of various tools and libraries for handling and analyzing bioimage data using DL techniques, such as bioimageio.core, core-bioimage-io-java, and imagej-modelzoo. The Zoo provides a user-friendly web application, BioEngine, for quick evaluation of models and supports interoperability and reproducibility. It is expected to promote rapid dissemination of DL developments, enrich and support advanced bioimage analysis workflows, and adhere to FAIR principles.