Avoiding a replication crisis in deep-learning-based bioimage analysis
Technologies: BioImage Model Zoo and ZeroCostDL4Mic
Perspective published in Nature methods, January 2021
Publisher: Nature Publishing Group US New York
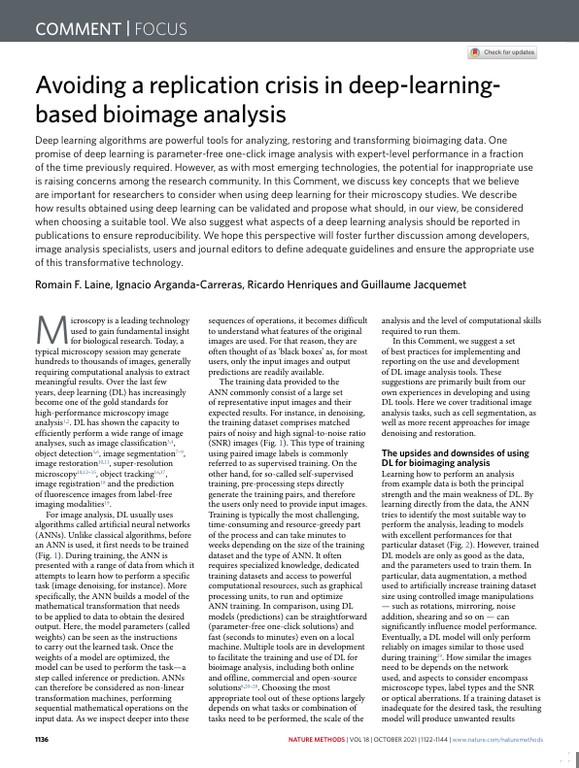
The manuscript explores the application of deep learning (DL) algorithms in microscopy image analysis and discusses the potential benefits and concerns. DL has shown promising results in various image analyses, including denoising, segmentation, and super-resolution microscopy. However, the lack of transparency and understanding of its limitations, particularly for generalizability, has raised concerns. The authors suggest several best practices for researchers using DL for bioimage analysis, such as validating results, choosing suitable tools, and reporting aspects of the analysis for reproducibility. They emphasize the importance of assessing the performance of DL models on new data and comparing predictions to ground-truth images using various metrics.