Technology
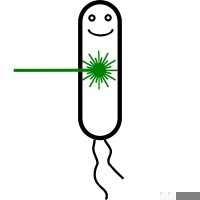
DeepAutoFocus
GitHub:
- HoldenLab/DeepAutoFocus
Publication: Whitley et al. Nature Communications 2021
- 66
DeepAutoFocus is an image-based autofocusing technique developed to enable long-term imaging experiments. It works by using infrared brightfield images of the sample and cross-correlation analysis to detect and compensate for focus drift during timelapse experiments.
In traditional microscopy, keeping the sample in focus over long periods of time is challenging due to thermal fluctuations and mechanical instabilities in the equipment. These issues cause the sample position to drift in and out of the focal plane, leading to blurring and loss of resolution in the images. Autofocus systems are therefore critical for acquiring high-quality, long-term timelapse data.
Most autofocus techniques rely on dedicated hardware components to measure the sample position, for example by reflecting an infrared beam off the sample coverslip. However, these methods fail when using more complex sample environments like microfluidics, where the additional interfaces interfere with the reflection signal.
DeepAutoFocus takes a different approach by using only the microscope camera to measure focus drift, removing the need for any extra hardware. It works by acquiring infrared brightfield images alongside every fluorescence image captured during the experiment. These images contain intrinsic focus information based on the sharpness of features in the image. By comparing new infrared images to a set of reference images, DeepAutoFocus can detect defocus and correct it by moving the sample stage.
In more detail:
-
A z-stack of infrared brightfield images is acquired at the start while ensuring the sample is in focus. This z-stack encompasses the full range of focus positions, both above and below best focus.
-
These reference images are used to build a focus curve that relates the cross-correlation value between images to the distance from best focus. The cross-correlation compares the similarity of two images, with the highest value occurring when the images are identical.
-
During the timelapse acquisition, a new infrared brightfield image is captured alongside every fluorescence image.
-
Cross-correlation maps are calculated between this new infrared image and the reference z-stack from step 1. This gives a cross-correlation value for each focus position in the z-stack.
-
The focus curve from step 2 allows translating these cross-correlation values into physical distances from best focus. The curve is empirically calibrated to account for differences between image sharpness and true focus position.
-
If the new image is calculated to be above or below best focus, the sample stage is moved to compensate and restore focus. This keeps every fluorescence image in focus despite any drift or fluctuations.
The key advantages of DeepAutoFocus are that it requires no additional hardware and provides focus stability in situations where traditional methods fail. By using images already being captured for data analysis, it also minimizes photobleaching and phototoxicity. The ability to stabilize focus for long durations enables new experiments and quantitative analyses previously impossible without complex active stabilization systems.
While the original implementation used infrared brightfield images, the concept of DeepAutoFocus can be applied to other modalities like phase contrast or DIC. The focus curve can also be tailored to different camera and objective combinations. Together with its flexibility for different sample environments, this makes DeepAutoFocus a versatile autofocus platform for automated long-term imaging across many areas of microscopy.
Publications featuring DeepAutoFocus
![]() |
FtsZ treadmilling is essential for Z-ring condensation and septal constriction initiation in Bacillus subtilis cell division Kevin D. Whitley, Calum Jukes, Nicholas Tregidgo, Eleni Karinou, Pedro Almada, Yann Cesbron, Ricardo Henriques, Cees Dekker, Séamus Holden Paper published in Nature Communications, April 2021 Technologies: CARE and DeepAutoFocus Funded by: BBSRC and Wellcome Trust News: Nanowerk DOI: 10.1038/s41467-021-22526-0 |
|