Technology
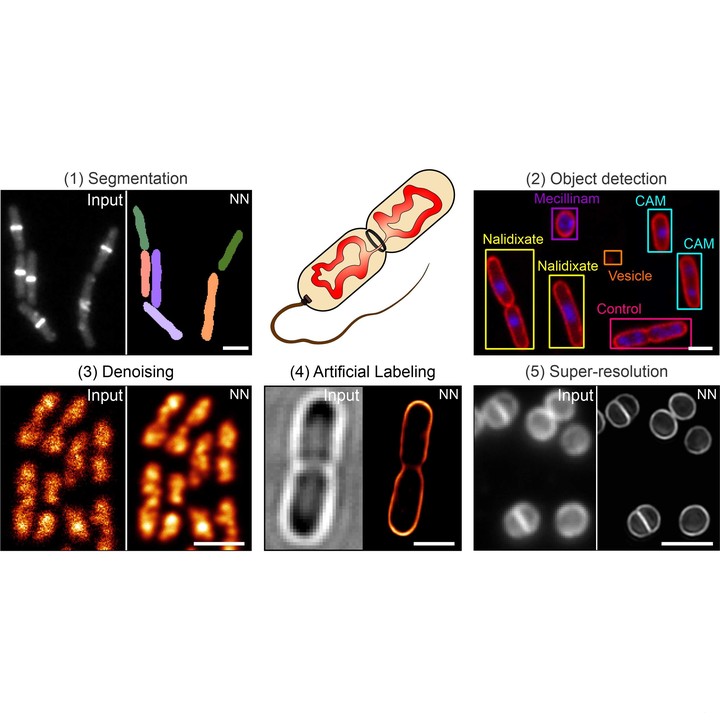
DeepBacs is an open-source deep learning framework for analyzing bacterial microscopy images. It utilizes state-of-the-art neural networks implemented on the ZeroCostDL4Mic platform to perform various image analysis tasks like segmentation, object detection, denoising, artificial labeling, and super-resolution prediction.
The key idea is to leverage existing deep learning models developed for eukaryotic cell analysis and adapt them for bacterial bioimaging. This avoids reinventing the wheel and allows microbiologists to take advantage of powerful AI tools.
The authors generated diverse labeled datasets of common model bacteria like E.coli, S.aureus, and B.subtilis imaged under different modalities - brightfield, fluorescence, confocal, and super-resolution microscopy. These were used to train neural networks for specific tasks.
For instance, the StarDist network was trained on brightfield and fluorescence images of cocci-shaped S.aureus cells to accurately segment and count individual cells, even at high densities. Similarly, the pix2pix generative adversarial network was trained to denoise low signal-to-noise ratio live cell movies of fluorescently labeled E.coli nucleoids, enabling longer imaging with 20x lower photobleaching.
Object detection using YOLOv2 network was shown to identify different bacterial growth phases and antibiotic-induced morphological phenotypes. And the fnet network generated artificial fluorescence images of E.coli cell membranes from label-free brightfield images.
By curating bacterial image datasets and providing pretrained models, DeepBacs allows microbiologists to quickly analyze their data without needing AI expertise. The modular and user-friendly design makes it easy to train custom models via transfer learning.
The open-source and cloud-based nature ensures wide accessibility and democratization. Overall, DeepBacs demonstrates how state-of-the-art deep learning can augment bacterial bioimaging and cell analysis with high accuracy, throughput and multiplexing. It has the potential to greatly benefit microbiology and antibiotic research.
Publications featuring DeepBacs
![]() |
The nucleoid of rapidly growing Escherichia coli localizes close to the inner membrane and is organized by transcription, translation, and cell geometry Christoph Spahn, Stuart Middlemiss, Estibaliz Gómez-de-Mariscal, Ricardo Henriques, Helge B. Bode, Séamus Holden, Mike Heilemann Paper published in Nature Communications, April 2025 Technologies: CARE, DeepBacs and ZeroCostDL4Mic Funded by: EMBO, ERC, H2021 and H2022 Blogs: BPoD - Biomedical Picture of the Day DOI: 10.1038/s41467-025-58723-4 |
|
![]() |
ReScale4DL - Balancing Pixel and Contextual Information for Enhanced Bioimage Segmentation Mariana G. Ferreira, Bruno M. Saraiva, António D. Brito, Mariana G. Pinho, Ricardo Henriques, Estibaliz Gómez-de-Mariscal Preprint published in bioRxiv, April 2025 Technologies: BioImage Model Zoo, DeepBacs, DL4MicEverywhere, NanoPyx, Rescale4DL and ZeroCostDL4Mic Funded by: CZI, EMBO, ERC, H2021 and H2022 DOI: 10.1101/2025.04.09.647871 |
|
![]() |
Harnessing artificial intelligence to reduce phototoxicity in live imaging Estibaliz Gómez-de-Mariscal, Mario Del Rosario, Joanna W. Pylvänäinen, Guillaume Jacquemet, Ricardo Henriques Perspective published in Journal of Cell Science, February 2024 Technologies: BioImage Model Zoo, CARE, DeepBacs, NanoJ-eSRRF, NanoJ-SQUIRREL, NanoJ-SRRF and ZeroCostDL4Mic Funded by: CZI, EMBO, ERC, H2021 and H2022 DOI: 10.1242/jcs.261545 |
|
![]() |
Transertion and cell geometry organize the Escherichia coli nucleoid during rapid growth Christoph Spahn, Stuart Middlemiss, Estibaliz Gómez-de-Mariscal, Ricardo Henriques, Helge B. Bode, Séamus Holden, Mike Heilemann Preprint published in bioRxiv, October 2023 Technologies: CARE, DeepAutoFocus, DeepBacs and ZeroCostDL4Mic Funded by: EMBO, ERC, H2021 and H2022 DOI: 10.1101/2023.10.16.562172 |
|
![]() |
Live-cell imaging in the deep learning era Joanna W Pylvänäinen, Estibaliz Gómez-de-Mariscal, Ricardo Henriques, Guillaume Jacquemet Review published in Current Opinion in Cell Biology, January 2023 Technologies: BioImage Model Zoo, DeepBacs, Fast4DReg, NanoJ, NanoJ-eSRRF, NanoJ-Fluidics and ZeroCostDL4Mic Funded by: CZI, EMBO, ERC and H2021 DOI: 10.1016/j.ceb.2023.102271 |
|
![]() |
DeepBacs for multi-task bacterial image analysis using open-source deep learning approaches Christoph Spahn, Estibaliz Gómez-de-Mariscal, Romain F. Laine, Pedro M. Pereira, Lucas von Chamier, Mia Conduit, Mariana G. Pinho, Guillaume Jacquemet, Séamus Holden, Mike Heilemann, Ricardo Henriques Paper published in Communications Biology, July 2022 Technologies: BioImage Model Zoo, CARE, DeepBacs, NanoJ, NanoJ-SQUIRREL and ZeroCostDL4Mic Funded by: CZI, ERC, FCT and Wellcome Trust DOI: 10.1038/s42003-022-03634-z |
|