Technology
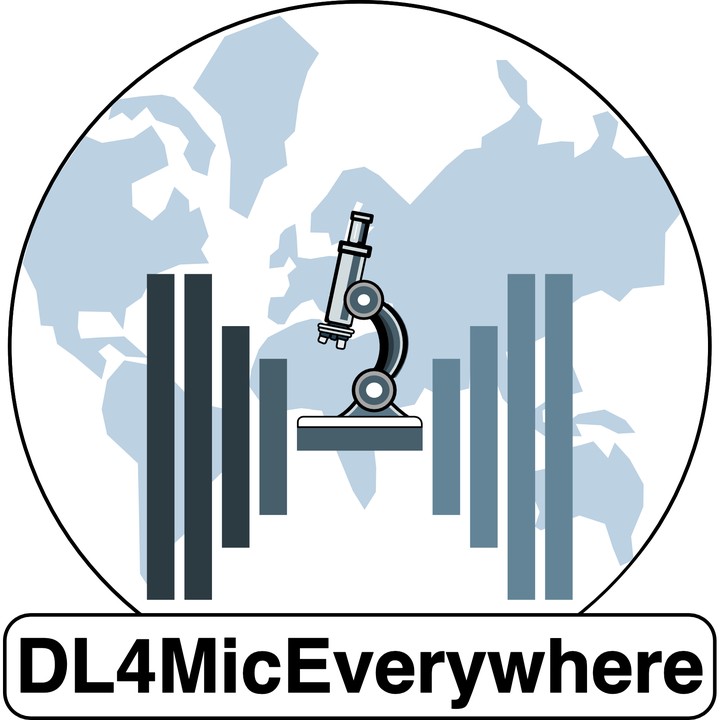
DL4MicEverywhere
GitHub:
- HenriquesLab/DL4MicEverywhere
Publication: Hidalgo-Cenalmor et al. Nature Methods 2024
8
DL4MicEverywhere is an open-source platform that aims to make deep learning more accessible for bioimage analysis. It builds on the previous ZeroCostDL4Mic platform and advances it further to allow more flexible training and deployment of deep learning models across diverse computational environments.
The key goal of DL4MicEverywhere is to democratize deep learning for microscopy image analysis by tackling some of the main barriers to adoption. Many researchers lack access to sufficiently large, annotated training datasets that are required to develop accurate deep learning models. Additionally, training these complex neural networks requires significant computational resources like GPUs which are expensive. There is also a general lack of expertise among biomedical researchers in designing, training and deploying deep neural networks.
To address these challenges, DL4MicEverywhere encapsulates various state-of-the-art deep learning solutions for bioimage analysis into easy to use Docker containers. This allows flexible training and deployment across laptops, workstations, clusters and cloud platforms without needing to install any dependencies. Pre-trained models are also shared to allow researchers to quickly get started analyzing their data. The platform lowers barriers to entry by abstracting away unnecessary complexity in model development.
A key emphasis in DL4MicEverywhere is on transparency, reproducibility and convenience. The self-contained Docker images ensure consistency across operating systems. Detailed documentation with usage tutorials facilitates adoption. Importantly, the open-source ethos enables community review, improvement and extension of the available methods. Researchers are encouraged to containerize their own models and contribute them back to grow the platform.
Publications featuring DL4MicEverywhere
![]() |
PhotoFiTT - A Quantitative Framework for Assessing Phototoxicity in Live-Cell Microscopy Experiments Mario Del Rosario, Estibaliz Gómez-de-Mariscal, Leonor Morgado, Raquel Portela, Guillaume Jacquemet, Pedro M. Pereira, Ricardo Henriques Preprint published in bioRxiv, July 2024 Technologies: DL4MicEverywhere, PhotoFiTT and ZeroCostDL4Mic Funded by: CZI, EMBO, ERC, FCT, H2021 and H2022 DOI: 10.1101/2024.07.16.603046 |
|
![]() |
DL4MicEverywhere - deep learning for microscopy made flexible, shareable and reproducible Iván Hidalgo-Cenalmor, Joanna W. Pylvänäinen, Mariana G. Ferreira, Craig T. Russell, Alon Saguy, Ignacio Arganda-Carreras, Yoav Shechtman, Guillaume Jacquemet, Ricardo Henriques, Estibaliz Gómez-de-Mariscal Paper published in Nature Methods, May 2024 Technologies: BioImage Model Zoo, DL4MicEverywhere and ZeroCostDL4Mic Funded by: EMBO, ERC, H2021 and H2022 News: Labonline, MSN, AZoRobotics and Aamuset Kaupunkimedia Blogs: news-medical.net DOI: 10.1038/s41592-024-02295-6 |
|