Democratising Live-Cell Adaptive Super-Resolution Microscopy based on SRRF
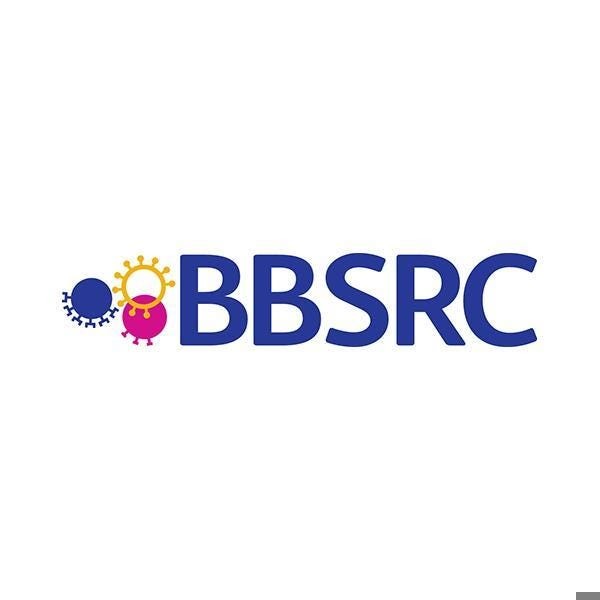
Type: Tools and Resources Development Fund
Principal Investigator: Ricardo Henriques
Investigators: Ricardo Henriques, Buzz Baum
Start-date: January 2019
End-date: February 2020
Human perception depends heavily on the capacity of the visual cortex to compensate for flaws in the structure of the eye. Evolutionarily, the architecture of the eye changed little over time while neuronal computation has taken on a major role in compensating for the optical limitations imposed by the `hardware' and optimising its performance under different conditions. Microscopy is following a similar shift. The basic optical design of microscopes has changed little over the last 20 years. However, we have achieved remarkable advancements in bypassing their physical limitations by finding new ways to collect and analyse images. Through computational-assisted approaches we are now able to achieve a massive increase in the resolution of these imaging systems (by 10-fold or more) and considerably reduce optical aberrations. Over a decade ago Super-Resolution Microscopy, a new field dedicated to increasing resolution in light microscopy, was conceived. In these techniques, methods such as PALM, STORM and SRRF, use advanced spatio-temporal analysis of the data-feed in microscopes to estimate the location of molecules, achieving a resolution near the molecular scale itself (<50nm). These technologies are revolutionizing biological and biomedical research. They allow us to observe for the first time the dynamic structure of cells at the nanoscale, beyond the capacity of any other method. Super-Resolution Microscopy is however limited by its complexity. The quality and resolution of these methods depends heavily on having considerable knowledge of the photophysics of the fluorophores used and of the characteristics of the sample being imaged. Non-artefactual high-resolution imaging depends on correctly exploiting these traits. Live-cell imaging entails a further degree of difficulty, as one needs to take also into account and minimize the toxic light burden imposed into cells by the existing methods. Here we propose iSRRF, a paradigm shift in Super-Resolution Microscopy where the decisions regarding imaging conditions are not determined by the researcher, but by an artificial intelligence engine that studies the sample and learns how to best optimize imaging. This computational approach will maximize the image quality and resolution while limiting the sample illumination to minimise live-cell phototoxicity. It will replace human intuition with empirical decisions based on mathematically well-defined actions. At its base, this approach will be based on the recently developed Super-Resolution Radial Fluctuations (SRRF) method, taking advantage of its capacity to enable live-cell Super-Resolution imaging in most modern light microscopes, even those not initially designed for Super-Resolution. SRRF is currently transforming the microscopy field, leading to the development of the first Super-Resolution cameras (iXon SRRF-Stream by Andor Technology). It is set to be integrated into the microscopes of most of the leading imaging companies. Following a similar model, iSRRF will be provided as an open-source, easy-to-use, easy-to-implement algorithm compatible with the extremely popular ImageJ and Micro-Manager image analysis and acquisition platforms, enabling the widest possible uptake both by companies and individual users. As a pilot project demonstrating iSRRF, we will study cell division. Cell division is often used as the benchmark for phototoxicity in microscopy as photodamage disrupts cell division, preventing it entirely if the damage is sufficiently high. It has, thus far, been a struggle to apply Super-Resolution Microscopy to study cell division due to the requirement for high laser illumination intensities when compared to conventional imaging methods. Our preliminary data shows however that iSRRF will be capable of following cell division over several hours while achieving a 2-to-10 fold increase in resolution. This capacity is beyond any other existing Super-Resolution method. Technical Summary Despite over 10-years of research on the Super-Resolution field, Super-Resolution microscopy remains fairly inaccessible to non-expert researchers and most biological problems. While in part this is due to a lack of access to Super-Resolution systems, it is dominantly because of a steep learning curve and inherent difficulty in setting up experiments compared conventional microscopy. Recently we have developed Super-Resolution Radial Fluctuations (SRRF - pronounced surf). SRRF allows microscopists to achieve optical resolutions of few tens of nanometres in live cells without causing meaningful light-induced damage. Where Super-Resolution light microscopy methods such as PALM, STORM and STED use intense sample illuminations that can disrupt the normal behaviour of the sample or even cause cell death, SRRF requires >100-fold less illumination. In parallel, we developed a second technology, SQUIRREL, as an approach to quantitatively map the quality and resolution of Super-Resolution images. SQUIRREL uses the robust assumption that Super-Resolution images should be higher-resolution representations of their diffraction-limited counterparts. Local deviations from this immediately highlight decay in image quality (i.e. image artefacts). By combining SRRF and SQUIRREL in a real-time analysis framework, it becomes possible to generate Super-Resolution images of known resolution and quality. This ability immediately opens the door to establish a machine learning approach that adapts the microscope acquisition and SRRF analysis settings to maximise these two values. This enables the microscopy system to: i) learn from the sample about how to improve imaging in real time, and ii) in a dynamic manner adapt to changes in the imaging properties of a living sample. With iSRRF, Super-Resolution will become considerably simpler and accessible to researchers. Achieving quality and resolution in images beyond the capacity of a system purely driven by a researcher.
Supported publications
![]() |
Closed mitosis requires local disassembly of the nuclear envelope Gautam Dey, Siân Culley, Scott Curran, Uwe Schmidt, Ricardo Henriques, Wanda Kukulski, Buzz Baum Paper published in Nature, August 2020 Technologies: CARE, NanoJ, NanoJ-SRRF and Nuclear-Pores as references Funded by: BBSRC and Wellcome Trust News: Nature Asia DOI: 10.1038/s41586-020-2648-3 |
|
![]() |
The cell biologist's guide to super-resolution microscopy Guillaume Jacquemet, Alexandre F. Carisey, Hellyeh Hamidi, Ricardo Henriques, Christophe Leterrier Review published in Journal of Cell Science, June 2020 Technologies: NanoJ, NanoJ-Fluidics, NanoJ-SRRF and ZeroCostDL4Mic Funded by: BBSRC and Wellcome Trust News: ScienMag and EurekAlert! DOI: 10.1242/jcs.240713 |
|
![]() |
Between life and death - strategies to reduce phototoxicity in super-resolution microscopy Kalina L Tosheva, Yue Yuan, Pedro Matos Pereira, Siân Culley, Ricardo Henriques Review published in Journal of Physics D - Applied Physics, January 2020 Technologies: CARE, NanoJ and NanoJ-Fluidics Funded by: BBSRC and Wellcome Trust DOI: 10.1088/1361-6463/ab6b95 |
|
![]() |
Automating multimodal microscopy with NanoJ-Fluidics Pedro Almada, Pedro M. Pereira, Siân Culley, Ghislaine Caillol, Fanny Boroni-Rueda, Christina L. Dix, Guillaume Charras, Buzz Baum, Romain F. Laine, Christophe Leterrier, Ricardo Henriques Paper published in Nature Communications, March 2019 Technologies: NanoJ, NanoJ-Fluidics, NanoJ-SQUIRREL, NanoJ-SRRF and NanoJ-VirusMapper Funded by: BBSRC and Wellcome Trust News: Technology Times, MSN, DNYUZ and Express Informer DOI: 10.1038/s41467-019-09231-9 |
|