Technology
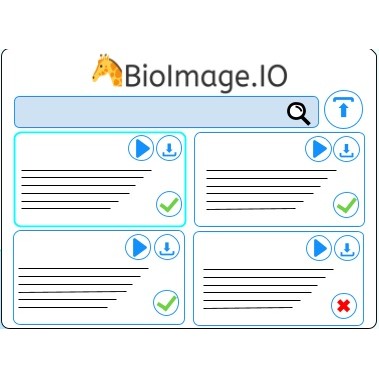
The BioImage Model Zoo is a community-driven resource that aims to make deep learning models for bioimage analysis more accessible, interoperable, and reusable. It provides a centralized platform for sharing standardized pre-trained models that can be easily deployed across different bioimage analysis software tools.
At the core of the BioImage Model Zoo is a standardized model description format that captures all the necessary metadata about a model, including details about the input and output data format, model architecture, pre-trained weight values, training data provenance, licensing, etc. This enables models from the Zoo to be portable across tools. The Zoo infrastructure and libraries handle automatically applying any prescribed pre- and post-processing steps defined for a model.
Models in the Zoo cover common bioimage analysis tasks like image segmentation, classification, restoration etc. for different imaging modalities. Each model comes bundled with example input and output data that users can test it on before deploying in their workflows.
The BioImage Model Zoo platform has three main components:
-
Model repository: This is built on top of Zenodo and holds all the models along with their description metadata. Each model gets a unique ID and DOI. Models can be contributed by anyone but go through a curation process before public hosting.
-
Website (bioimage.io): This provides the user-facing interface to search, browse and interact with models from the Zoo. Users can test models directly in the browser through the 'Test Run' interface powered by the BioEngine framework (see below). The website auto-generates an interactive model card for each model that summarizes its purpose, usage, training data etc.
-
Developer tools and infrastructure: The Zoo provides Python and Java libraries to standardize model consumption and deployment across tools. The 'bioimageio.core' Python library handles all the steps for ingesting a model from the Zoo, running inference, and post-processing outputs. The BioEngine framework enables browser-based deployment of models, allowing users to test them through web applications.
Additionally, the Zoo has partnered with several bioimage analysis tools like ilastik, QuPath, ImageJ, StarDist etc. to ensure seamless integration. These tools can directly access Zoo models using the standardized libraries and formats. The Zoo also stores links between models, their training datasets and notebooks to ensure transparency and reproducibility.
To contribute a model, users can upload its description metadata and weights to a Zenodo record through the bioimage.io website or programmatically using the Python developer libraries. The infrastructure automatically handles assigning a unique ID, testing the model, and integrating it into the Zoo through a curation process. The aim is to support FAIR (Findable, Accessible, Interoperable and Reusable) sharing of deep learning models for bioimage analysis.
The BioImage Model Zoo aims to accelerate the adoption of AI-based techniques in bioimage analysis by providing a community platform for sharing pre-trained models across tools. It handles all the complexities behind model standardization, testing, and deployment through a transparent infrastructure centered around the model metadata format. By integrating with multiple analysis software tools, it allows bench biologists to easily access state-of-the-art deep learning solutions.
Publications featuring BioImage Model Zoo
![]() |
ReScale4DL - Balancing Pixel and Contextual Information for Enhanced Bioimage Segmentation Mariana G. Ferreira, Bruno M. Saraiva, António D. Brito, Mariana G. Pinho, Ricardo Henriques, Estibaliz Gómez-de-Mariscal Preprint published in bioRxiv, April 2025 Technologies: BioImage Model Zoo, DeepBacs, DL4MicEverywhere, NanoPyx, Rescale4DL and ZeroCostDL4Mic Funded by: CZI, EMBO, ERC, H2021 and H2022 DOI: 10.1101/2025.04.09.647871 |
|
![]() |
DL4MicEverywhere - deep learning for microscopy made flexible, shareable and reproducible Iván Hidalgo-Cenalmor, Joanna W. Pylvänäinen, Mariana G. Ferreira, Craig T. Russell, Alon Saguy, Ignacio Arganda-Carreras, Yoav Shechtman, Guillaume Jacquemet, Ricardo Henriques, Estibaliz Gómez-de-Mariscal Paper published in Nature Methods, May 2024 Technologies: BioImage Model Zoo, DL4MicEverywhere and ZeroCostDL4Mic Funded by: EMBO, ERC, H2021 and H2022 News: Labonline, MSN, AZoRobotics and Aamuset Kaupunkimedia Blogs: news-medical.net DOI: 10.1038/s41592-024-02295-6 |
|
![]() |
Harnessing artificial intelligence to reduce phototoxicity in live imaging Estibaliz Gómez-de-Mariscal, Mario Del Rosario, Joanna W. Pylvänäinen, Guillaume Jacquemet, Ricardo Henriques Perspective published in Journal of Cell Science, February 2024 Technologies: BioImage Model Zoo, CARE, DeepBacs, NanoJ-eSRRF, NanoJ-SQUIRREL, NanoJ-SRRF and ZeroCostDL4Mic Funded by: CZI, EMBO, ERC, H2021 and H2022 DOI: 10.1242/jcs.261545 |
|
![]() |
CLEM-Reg - An automated point cloud based registration algorithm for correlative light and volume electron microscopy Daniel Krentzel, Matouš Elphick, Marie-Charlotte Domart, Christopher J. Peddie, Romain F. Laine, Ricardo Henriques, Lucy M. Collinson, Martin L. Jones Preprint published in bioRxiv, May 2023 Technologies: BioImage Model Zoo, CLEM-Reg and ZeroCostDL4Mic Funded by: CZI and ERC DOI: 10.1101/2023.05.11.540445 |
|
![]() |
Live-cell imaging in the deep learning era Joanna W Pylvänäinen, Estibaliz Gómez-de-Mariscal, Ricardo Henriques, Guillaume Jacquemet Review published in Current Opinion in Cell Biology, January 2023 Technologies: BioImage Model Zoo, DeepBacs, Fast4DReg, NanoJ, NanoJ-eSRRF, NanoJ-Fluidics and ZeroCostDL4Mic Funded by: CZI, EMBO, ERC and H2021 DOI: 10.1016/j.ceb.2023.102271 |
|
![]() |
Roadmap on deep learning for microscopy Giovanni Volpe, Carolina Wählby, Lei Tian, Michael Hecht, Artur Yakimovich, Kristina Monakhova, Laura Waller, Ivo F Sbalzarini, Christopher A Metzler, Mingyang Xie, Kevin Zhang, Isaac CD Lenton, Halina Rubinsztein-Dunlop, Daniel Brunner, Bijie Bai, Aydogan Ozcan, Daniel Midtvedt, Hao Wang, Nataša Sladoje, Joakim Lindblad, Jason T Smith, Marien Ochoa, Margarida Barroso, Xavier Intes, Tong Qiu, Li-Yu Yu, Sixian You, Yongtao Liu, Maxim A Ziatdinov, Sergei V Kalinin, Arlo Sheridan, Uri Manor, Elias Nehme, Ofri Goldenberg, Yoav Shechtman, Henrik K Moberg, Christoph Langhammer, Barbora Špačková, Saga Helgadottir, Benjamin Midtvedt, Aykut Argun, Tobias Thalheim, Frank Cichos, Stefano Bo, Lars Hubatsch, Jesus Pineda, Carlo Manzo, Harshith Bachimanchi, Erik Selander, Antoni Homs-Corbera, Martin Fränzl, Kevin de Haan, Yair Rivenson, Zofia Korczak, Caroline Beck Adiels, Mite Mijalkov, Dániel Veréb, Yu-Wei Chang, Joana B Pereira, Damian Matuszewski, Gustaf Kylberg, Ida-Maria Sintorn, Juan C Caicedo, Beth A Cimini, Muyinatu A Lediju Bell, Bruno M Saraiva, Guillaume Jacquemet, Ricardo Henriques, Wei Ouyang, Trang Le, Estibaliz Gómez-de-Mariscal, Daniel Sage, Arrate Muñoz-Barrutia, Ebba Josefson Lindqvist, Johanna Bergman Preprint published in arXiv, January 2023 Technologies: BioImage Model Zoo, CARE and ZeroCostDL4Mic Funded by: CZI, EMBO, ERC and H2021 DOI: 10.48550/arXiv.2303.03793 |
|
![]() |
DeepBacs for multi-task bacterial image analysis using open-source deep learning approaches Christoph Spahn, Estibaliz Gómez-de-Mariscal, Romain F. Laine, Pedro M. Pereira, Lucas von Chamier, Mia Conduit, Mariana G. Pinho, Guillaume Jacquemet, Séamus Holden, Mike Heilemann, Ricardo Henriques Paper published in Communications Biology, July 2022 Technologies: BioImage Model Zoo, CARE, DeepBacs, NanoJ, NanoJ-SQUIRREL and ZeroCostDL4Mic Funded by: CZI, ERC, FCT and Wellcome Trust DOI: 10.1038/s42003-022-03634-z |
|
![]() |
Bioimage model zoo - a community-driven resource for accessible deep learning in bioimage analysis Wei Ouyang, Fynn Beuttenmueller, Estibaliz Gómez-de-Mariscal, Constantin Pape, Tom Burke, Carlos Garcia-López-de-Haro, Craig Russell, Lucía Moya-Sans, Cristina de-la-Torre-Gutiérrez, Deborah Schmidt, Dominik Kutra, Maksim Novikov, Martin Weigert, Uwe Schmidt, Peter Bankhead, Guillaume Jacquemet, Daniel Sage, Ricardo Henriques, Arrate Muñoz-Barrutia, Emma Lundberg, Florian Jug, Anna Kreshuk Preprint published in BioRxiv, January 2022 Technologies: BioImage Model Zoo, CARE and ZeroCostDL4Mic Funded by: CZI, EMBO, ERC and H2021 DOI: 10.1101/2022.06.07.495102 |
|
![]() |
Avoiding a replication crisis in deep-learning-based bioimage analysis Romain F Laine, Ignacio Arganda-Carreras, Ricardo Henriques, Guillaume Jacquemet Perspective published in Nature methods, January 2021 Technologies: BioImage Model Zoo and ZeroCostDL4Mic Funded by: EMBO, ERC and Wellcome Trust DOI: 10.1038/s41592-021-01284-3 |
|
Funding contributing to BioImage Model Zoo
![]() |
SMALS - Smart Microscopy for Adaptative Live Super-resolution imaging to elucidate the initial steps of the HIV viral transmission Estibaliz Gómez-de-Mariscal Alias: SMALS Funded by: FCT - CEEC Individual Duration: April 2024 - March 2027 |
![]() |
Real-Time high-content Super-Resolution Imaging of ES Cell States Eran Meshorer, Ricardo Henriques, Anna Kreshuk, Sandrine Lévêque-Fort, Nicolas Bourg, Genevieve Almouzni Alias: RT-SuperES Funded by: H2022 - EIC Pathfinder Open Duration: April 2022 - March 2027 Publications: 15 |
![]() |
Artificial Intelligence for Image Data Analysis in the Life Sciences Anna Kreshuk, Florian Jug, Ricardo Henriques, Wei Ouyang, Arrate Muñoz-Barrutia, Emma Lundberg, Matthew Hartley Alias: AI4Life Funded by: H2021 - INFRA Duration: September 2021 - August 2025 Publications: 19 |