Technology
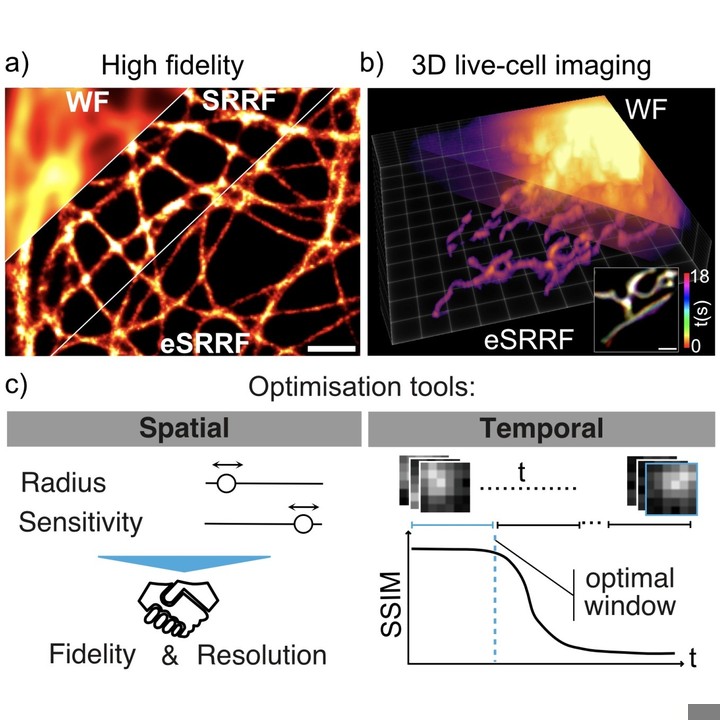
eSRRF, which stands for "enhanced super-resolution radial fluctuations", is an advanced fluorescence microscopy technique that can image biological structures at resolutions better than the diffraction limit of light. It builds upon and substantially improves an earlier method called SRRF (super-resolution radial fluctuations) by achieving higher image fidelity, resolution, and ease of use.
The basic principle behind eSRRF and SRRF is that small fluctuations in fluorescence intensity over time can be analyzed to reconstruct super-resolution images. This relies on the fact that when fluorescent labels like fluorescent proteins randomly switch between bright and dark states over time, the center positions of the blinking molecules can be determined with higher precision than the diffraction limit. eSRRF processes an image sequence to identify these intensity fluctuations at sub-diffraction length scales and uses this information to produce a super-resolved reconstruction.
In more detail, the eSRRF workflow is:
-
Acquire a time-series of diffraction-limited fluorescence microscopy images capturing intensity fluctuations from blinking fluorophores. Hundreds to thousands of frames are usually needed depending on the sample.
-
Upscale each frame by interpolation to increase sampling. This minimizes pixelation artifacts in later steps.
-
Calculate the intensity gradient at each pixel to determine locations of signal change. This captures fluctuations occurring within the point spread function.
-
Generate a radiality weighting map based on the user-defined analysis radius. This weights pixels according to their distance from the current pixel.
-
Combine the gradient and radiality maps to yield a "radial gradient convergence" (RGC) map describing local fluctuations. Each pixel has an RGC time trace over the image sequence.
-
Perform temporal analysis like autocorrelation or cross-correlation on the RGC traces to extract fluctuations occurring at different time intervals. This yields a super-resolution reconstruction with enhanced resolution.
Compared to the original SRRF implementation, eSRRF substantially improves the RGC calculation and overall workflow. Key innovations include:
- New Fourier interpolation to minimize pixelation
- RGC estimation considers local pixel neighborhoods defined by the radiality map instead of just discrete points
- Automated parameter optimization based on resolution and image quality metrics
- Assesses the best temporal analysis frames for reconstructing dynamics
- Extends SRRF to 3D by combining with multifocus microscopy
Together, these advances provide higher-fidelity super-resolution images across varied sample types while minimizing artifacts and user bias. eSRRF makes the SRRF principle more robust and accessible for live-cell nanoscale imaging.
The eSRRF method has been demonstrated to work across different microscopy modalities like TIRF, light sheet, spinning disk confocal, and others. It can improve resolution ~2-fold laterally and axially when applied to 3D multifocus data. The enhanced performance over SRRF has been shown quantitatively on calibration standards and simulated data. Overall, eSRRF sets new standards for optimization and information extraction to move super-resolution microscopy toward more reliable and unbiased analyses.
Publications featuring NanoJ-eSRRF
![]() |
Expansion and fluctuations-enhanced microscopy for nanoscale molecular profiling of cells and tissues Dominik Kylies, Hannah S. Heil, Arturo G. Vesga, Mario Del Rosario, Maria Schwerk, Malte Kuehl, Milagros N. Wong, Victor G. Puelles, Ricardo Henriques Paper published in Nature Protocols, July 2025 Technologies: NanoJ (), NanoJ-eSRRF (), NanoJ-SQUIRREL (), NanoJ-SRRF (), NanoPyx () and Nuclear-Pores as references Funded by: CZI, EMBO, ERC, FCT, H2021 and H2022 DOI: 10.1038/s41596-025-01178-0 |
|
![]() |
Nanoscale imaging of biological systems via expansion and super-resolution microscopy Daria Aristova, Dominik Kylies, Mario Del Rosario, Hannah S. Heil, Maria Schwerk, Malte Kuehl, Milagros N. Wong, Ricardo Henriques, Victor G. Puelles Paper published in Applied Physics Reviews, April 2025 Technologies: NanoJ-eSRRF () and NanoJ-SRRF () Funded by: CZI, EMBO, ERC, FCT, H2021 and H2022 DOI: 10.1063/5.0240464 |
|
![]() |
Efficiently accelerated bioimage analysis with NanoPyx, a Liquid Engine-powered Python framework Bruno M. Saraiva, Inês Cunha, António D. Brito, Gautier Follain, Raquel Portela, Robert Haase, Pedro M. Pereira, Guillaume Jacquemet, Ricardo Henriques Paper published in Nature Methods, January 2025 Technologies: Fast4DReg (), NanoJ (), NanoJ-eSRRF (), NanoJ-SQUIRREL () and NanoPyx () Funded by: CZI, EMBO, ERC, FCT, H2021 and H2022 News: Tempo.pt, Nouvelles du monde and RTP DOI: 10.1038/s41592-024-02562-6 |
|
![]() |
Harnessing artificial intelligence to reduce phototoxicity in live imaging Estibaliz Gómez-de-Mariscal, Mario Del Rosario, Joanna W. Pylvänäinen, Guillaume Jacquemet, Ricardo Henriques Perspective published in Journal of Cell Science, February 2024 Technologies: BioImage Model Zoo (), CARE (), DeepBacs (), NanoJ-eSRRF (), NanoJ-SQUIRREL (), NanoJ-SRRF () and ZeroCostDL4Mic () Funded by: CZI, EMBO, ERC, H2021 and H2022 DOI: 10.1242/jcs.261545 |
|
![]() |
High-fidelity 3D live-cell nanoscopy through data-driven enhanced super-resolution radial fluctuation Romain F. Laine, Hannah S. Heil, Simao Coelho, Jonathon Nixon-Abell, Angélique Jimenez, Theresa Wiesner, Damián Martínez, Tommaso Galgani, Louise Régnier, Aki Stubb, Gautier Follain, Samantha Webster, Jesse Goyette, Aurelien Dauphin, Audrey Salles, Siân Culley, Guillaume Jacquemet, Bassam Hajj, Christophe Leterrier, Ricardo Henriques Paper published in Nature Methods, November 2023 Technologies: CARE (), NanoJ (), NanoJ-eSRRF (), NanoJ-SQUIRREL (), NanoJ-SRRF () and Nuclear-Pores as references Funded by: CZI, EMBO, ERC, FCT, H2021, H2022, InnOValley and Wellcome Trust News: Photonics.com, The Science Times, Optics.org and Phys.org Blogs: Springer Nature Protocols and Methods Community DOI: 10.1038/s41592-023-02057-w |
|
Funding contributing to NanoJ-eSRRF
![]() |
Cutting-edge super-resolution image analysis in napari through NanoJ Bruno Saraiva, Ricardo Henriques Funded by: CZI - Applications - napari Plugin Foundations grants Duration: January 2023 - December 2023 Publications: 4 |