Technology
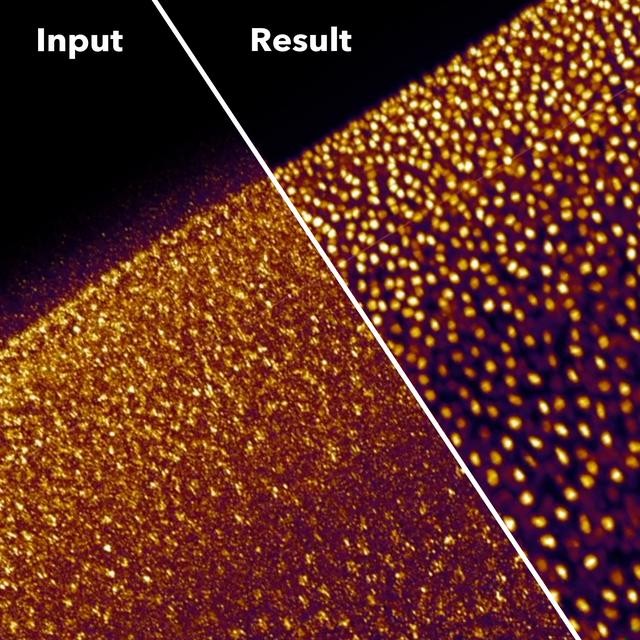
CARE (Content-Aware image Restoration) is a deep learning based approach for restoring and enhancing fluorescence microscopy images. It uses convolutional neural networks to learn complex mappings between low quality input images and higher quality target images, allowing the restoration of images beyond the physical limits of the microscope.
CARE networks are trained on pairs of images depicting the same content - one image acquired under suboptimal conditions (low signal-to-noise ratio, low resolution etc.) serves as the input, while the other image shows the desired target quality. The type of restoration determines what constitutes the input and target images. For example, for image denoising the input could be a low laser power acquisition exhibiting high noise, while the target is a clean image obtained with higher laser power.
The pairs of input and target images showing the same content are used to train a neural network to perform the restoration mapping. While creating such training data is typically difficult for image processing tasks, the authors of CARE introduce several clever strategies to obtain suitable data without extensive manual labor:
1) For denoising, they interleave low and high laser power acquisitions during imaging to obtain registered image pairs of the same sample.
2) For isotropic resolution restoration, they use the higher resolved lateral planes of a volume as target and synthetically generate matching axial planes with lower resolution.
3) For super-resolution, they create simulated ground truth images of structures of interest and realistically degrade them to yield synthetic input images.
Using these strategies, thousands of aligned input-target pairs showing diverse biological image contents are generated. A convolutional neural network with a U-Net-like architecture is then trained to restore low quality inputs to match the target images. The particular network architecture and training process is tailored to each specific restoration type.
Once the CARE network is trained, it can be applied to new unseen microscopy images that were acquired under suboptimal conditions in order to computationally restore and enhance image quality beyond the physical limits of the microscope.
Some concrete examples demonstrated:
-
Denoising time-lapse recordings of live samples at very high frame rates, since a CARE network can restore the individual noisy frames to a quality otherwise only achieved through longer exposure or higher laser power not compatible with living samples
-
Projection of fluorescently labeled cell layers from noisy 3D volumes at high speed, by using a network trained to jointly perform projection and denoising
-
Isotropic restoration of anisotropic volumes through a network that learns to computationally restore missing axial resolution from lateral planes
-
Super-resolution enhancement of microtubules and secretory granules from widefield microscopy through a network trained only on simulated structures
CARE leverages recent advances in deep learning to computationally improve microscopy images beyond the physical limits of the respective microscope. It allows trading off imaging parameters that ordinarily cannot be optimized jointly, thereby enhancing observable biological phenomena. Through tailored training data generation and network design, CARE delivers marked improvements in image quality for a variety of restoration problems in fluorescence microscopy.
Publications featuring CARE
![]() |
The nucleoid of rapidly growing Escherichia coli localizes close to the inner membrane and is organized by transcription, translation, and cell geometry Christoph Spahn, Stuart Middlemiss, Estibaliz Gómez-de-Mariscal, Ricardo Henriques, Helge B. Bode, Séamus Holden, Mike Heilemann Paper published in Nature Communications, April 2025 Technologies: CARE, DeepBacs and ZeroCostDL4Mic Funded by: EMBO, ERC, H2021 and H2022 Blogs: BPoD - Biomedical Picture of the Day DOI: 10.1038/s41467-025-58723-4 |
|
![]() |
Harnessing artificial intelligence to reduce phototoxicity in live imaging Estibaliz Gómez-de-Mariscal, Mario Del Rosario, Joanna W. Pylvänäinen, Guillaume Jacquemet, Ricardo Henriques Perspective published in Journal of Cell Science, February 2024 Technologies: BioImage Model Zoo, CARE, DeepBacs, NanoJ-eSRRF, NanoJ-SQUIRREL, NanoJ-SRRF and ZeroCostDL4Mic Funded by: CZI, EMBO, ERC, H2021 and H2022 DOI: 10.1242/jcs.261545 |
|
![]() |
CellTracksColab is a platform that enables compilation, analysis, and exploration of cell tracking data Estibaliz Gómez-de-Mariscal, Hanna Grobe, Joanna W Pylvänäinen, Laura Xénard, Ricardo Henriques, Jean-Yves Tinevez, Guillaume Jacquemet Published in PLOS Biology, January 2024 Technologies: CARE, CellTracksColab and ZeroCostDL4Mic DOI: 10.1371/journal.pbio.3002740 |
|
![]() |
Transertion and cell geometry organize the Escherichia coli nucleoid during rapid growth Christoph Spahn, Stuart Middlemiss, Estibaliz Gómez-de-Mariscal, Ricardo Henriques, Helge B. Bode, Séamus Holden, Mike Heilemann Preprint published in bioRxiv, October 2023 Technologies: CARE, DeepAutoFocus, DeepBacs and ZeroCostDL4Mic Funded by: EMBO, ERC, H2021 and H2022 DOI: 10.1101/2023.10.16.562172 |
|
![]() |
Fast4DReg–fast registration of 4D microscopy datasets Joanna W Pylvänäinen, Romain F Laine, Bruno MS Saraiva, Sujan Ghimire, Gautier Follain, Ricardo Henriques, Guillaume Jacquemet Paper published in Journal of Cell Science, January 2023 Technologies: CARE, Fast4DReg, NanoJ and ZeroCostDL4Mic Funded by: CZI and ERC DOI: 10.1242/jcs.260728 |
|
![]() |
Roadmap on deep learning for microscopy Giovanni Volpe, Carolina Wählby, Lei Tian, Michael Hecht, Artur Yakimovich, Kristina Monakhova, Laura Waller, Ivo F Sbalzarini, Christopher A Metzler, Mingyang Xie, Kevin Zhang, Isaac CD Lenton, Halina Rubinsztein-Dunlop, Daniel Brunner, Bijie Bai, Aydogan Ozcan, Daniel Midtvedt, Hao Wang, Nataša Sladoje, Joakim Lindblad, Jason T Smith, Marien Ochoa, Margarida Barroso, Xavier Intes, Tong Qiu, Li-Yu Yu, Sixian You, Yongtao Liu, Maxim A Ziatdinov, Sergei V Kalinin, Arlo Sheridan, Uri Manor, Elias Nehme, Ofri Goldenberg, Yoav Shechtman, Henrik K Moberg, Christoph Langhammer, Barbora Špačková, Saga Helgadottir, Benjamin Midtvedt, Aykut Argun, Tobias Thalheim, Frank Cichos, Stefano Bo, Lars Hubatsch, Jesus Pineda, Carlo Manzo, Harshith Bachimanchi, Erik Selander, Antoni Homs-Corbera, Martin Fränzl, Kevin de Haan, Yair Rivenson, Zofia Korczak, Caroline Beck Adiels, Mite Mijalkov, Dániel Veréb, Yu-Wei Chang, Joana B Pereira, Damian Matuszewski, Gustaf Kylberg, Ida-Maria Sintorn, Juan C Caicedo, Beth A Cimini, Muyinatu A Lediju Bell, Bruno M Saraiva, Guillaume Jacquemet, Ricardo Henriques, Wei Ouyang, Trang Le, Estibaliz Gómez-de-Mariscal, Daniel Sage, Arrate Muñoz-Barrutia, Ebba Josefson Lindqvist, Johanna Bergman Preprint published in arXiv, January 2023 Technologies: BioImage Model Zoo, CARE and ZeroCostDL4Mic Funded by: CZI, EMBO, ERC and H2021 DOI: 10.48550/arXiv.2303.03793 |
|
![]() |
DeepBacs for multi-task bacterial image analysis using open-source deep learning approaches Christoph Spahn, Estibaliz Gómez-de-Mariscal, Romain F. Laine, Pedro M. Pereira, Lucas von Chamier, Mia Conduit, Mariana G. Pinho, Guillaume Jacquemet, Séamus Holden, Mike Heilemann, Ricardo Henriques Paper published in Communications Biology, July 2022 Technologies: BioImage Model Zoo, CARE, DeepBacs, NanoJ, NanoJ-SQUIRREL and ZeroCostDL4Mic Funded by: CZI, ERC, FCT and Wellcome Trust DOI: 10.1038/s42003-022-03634-z |
|
![]() |
Bioimage model zoo - a community-driven resource for accessible deep learning in bioimage analysis Wei Ouyang, Fynn Beuttenmueller, Estibaliz Gómez-de-Mariscal, Constantin Pape, Tom Burke, Carlos Garcia-López-de-Haro, Craig Russell, Lucía Moya-Sans, Cristina de-la-Torre-Gutiérrez, Deborah Schmidt, Dominik Kutra, Maksim Novikov, Martin Weigert, Uwe Schmidt, Peter Bankhead, Guillaume Jacquemet, Daniel Sage, Ricardo Henriques, Arrate Muñoz-Barrutia, Emma Lundberg, Florian Jug, Anna Kreshuk Preprint published in BioRxiv, January 2022 Technologies: BioImage Model Zoo, CARE and ZeroCostDL4Mic Funded by: CZI, EMBO, ERC and H2021 DOI: 10.1101/2022.06.07.495102 |
|
![]() |
Democratising deep learning for microscopy with ZeroCostDL4Mic Lucas von Chamier, Romain F. Laine, Johanna Jukkala, Christoph Spahn, Daniel Krentzel, Elias Nehme, Martina Lerche, Sara Hernández-Pérez, Pieta K. Mattila, Eleni Karinou, Séamus Holden, Ahmet Can Solak, Alexander Krull, Tim-Oliver Buchholz, Martin L. Jones, Loïc A. Royer, Christophe Leterrier, Yoav Shechtman, Florian Jug, Mike Heilemann, Guillaume Jacquemet, Ricardo Henriques Paper published in Nature Communications, April 2021 Technologies: CARE, NanoJ, NanoJ-SQUIRREL, NanoJ-SRRF and ZeroCostDL4Mic Funded by: EMBO, ERC and Wellcome Trust News: AZO Life Sciences, Drug Target Review, Nanotechnology Now and The Medical News Blogs: Microbiome Digest - Bik's Picks DOI: 10.1038/s41467-021-22518-0 |
|
![]() |
The field guide to 3D printing in microscopy Mario Del Rosario, Hannah S Heil, Afonso Mendes, Vittorio Saggiomo, Ricardo Henriques Review published in Adv. Biol., January 2021 Technologies: CARE, NanoJ, NanoJ-Fluidics and NanoJ-SRRF Funded by: EMBO, ERC and Wellcome Trust DOI: 10.1002/adbi.202100994 |
|
![]() |
Closed mitosis requires local disassembly of the nuclear envelope Gautam Dey, Siân Culley, Scott Curran, Uwe Schmidt, Ricardo Henriques, Wanda Kukulski, Buzz Baum Paper published in Nature, August 2020 Technologies: CARE, NanoJ, NanoJ-SRRF and Nuclear-Pores as references Funded by: BBSRC and Wellcome Trust News: Nature Asia DOI: 10.1038/s41586-020-2648-3 |
|
![]() |
Between life and death - strategies to reduce phototoxicity in super-resolution microscopy Kalina L Tosheva, Yue Yuan, Pedro Matos Pereira, Siân Culley, Ricardo Henriques Review published in Journal of Physics D - Applied Physics, January 2020 Technologies: CARE, NanoJ and NanoJ-Fluidics Funded by: BBSRC and Wellcome Trust DOI: 10.1088/1361-6463/ab6b95 |
|
![]() |
An Introduction to Live-Cell Super-Resolution Imaging Siân Culley, Pedro Matos Pereira, Romain F Laine, Ricardo Henriques Book chapter published in Imaging from Cells to Animals In Vivo, January 2020 Technologies: CARE, NanoJ, NanoJ-Fluidics, NanoJ-SQUIRREL and QuickPALM DOI: 10.1201/9781315174662-4 |
|
![]() |
Artificial intelligence for microscopy - what you should know Lucas von Chamier, Romain F. Laine, Ricardo Henriques Review published in Biochemical Society Transactions, July 2019 Technologies: CARE, NanoJ, NanoJ-Fluidics, NanoJ-SQUIRREL and NanoJ-SRRF Funded by: BBSRC and Wellcome Trust News: Azooptics.com DOI: 10.1042/bst20180391 |
|
![]() |
NanoJ - a high-performance open-source super-resolution microscopy toolbox Romain F Laine, Kalina L Tosheva, Nils Gustafsson, Robert D M Gray, Pedro Almada, David Albrecht, Gabriel T Risa, Fredrik Hurtig, Ann-Christin Lindås, Buzz Baum, Jason Mercer, Christophe Leterrier, Pedro M Pereira, Siân Culley, Ricardo Henriques Paper published in Journal of Physics D - Applied Physics, January 2019 Technologies: CARE, NanoJ, NanoJ-Fluidics, NanoJ-SQUIRREL, NanoJ-SRRF, NanoJ-VirusMapper and QuickPALM Funded by: BBSRC and Wellcome Trust DOI: 10.1088/1361-6463/ab0261 |
|
![]() |
Content-aware image restoration - pushing the limits of fluorescence microscopy Martin Weigert, Uwe Schmidt, Tobias Boothe, Andreas Müller, Alexandr Dibrov, Akanksha Jain, Benjamin Wilhelm, Deborah Schmidt, Coleman Broaddus, Siân Culley, Mauricio Rocha-Martins, Fabián Segovia-Miranda, Caren Norden, Ricardo Henriques, Marino Zerial, Michele Solimena, Jochen Rink, Pavel Tomancak, Loic Royer, Florian Jug, Eugene W. Myers Paper published in Nature Methods, November 2018 Technologies: CARE and NanoJ-SQUIRREL Funded by: BBSRC and Wellcome Trust News: Technology Networks, VBIO, Innovations Report and Informationsdienst Wissenschaft DOI: 10.1038/s41592-018-0216-7 |
|
Funding contributing to CARE
![]() |
SMALS - Smart Microscopy for Adaptative Live Super-resolution imaging to elucidate the initial steps of the HIV viral transmission Estibaliz Gómez-de-Mariscal Alias: SMALS Funded by: FCT - CEEC Individual Duration: April 2024 - March 2027 |
![]() |
3D Nanoscope - a highly accessible, high-performance device for live cell nanoscopy Arturo G. Vesga Alias: 3DNanoScope4All Funded by: Marie Curie - HORIZON TMA MSCA Postdoctoral Fellowships Duration: March 2024 - February 2026 |
![]() |
Sub-cellular Metabolic Compartmentalization During Oocyte Development Zita Carvalho dos Santos, Ricardo Henriques, Jorge Carvalho Funded by: CZI - Measuring Metabolism Across Scales Duration: January 2024 - December 2026 |
![]() |
Decoding T cell receptor signalling and membrane topology Simao Coelho Funded by: FCT - Concurso Estímulo ao Emprego Científico Duration: July 2022 - June 2028 Publications: 1 |
![]() |
Real-Time high-content Super-Resolution Imaging of ES Cell States Eran Meshorer, Ricardo Henriques, Anna Kreshuk, Sandrine Lévêque-Fort, Nicolas Bourg, Genevieve Almouzni Alias: RT-SuperES Funded by: H2022 - EIC Pathfinder Open Duration: April 2022 - March 2027 Publications: 15 |
![]() |
VP-CLEM-KIT - a pipeline for democratising volumetric visual proteomics Lucy Collinson, Ricardo Henriques, Paul French Funded by: CZI - Visual Proteomics Imaging Duration: December 2021 - June 2024 Publications: 18 |
![]() |
Enabling Live-Cell 4D Super-Resolution Microscopy Guided by Artificial Intelligence Ricardo Henriques Alias: SelfDriving4DSR Funded by: ERC - Consolidator Duration: September 2021 - September 2026 Publications: 34 |
![]() |
Mapping the early stages of HIV-1 infection by live-cell 4D Super-Resolution Microscopy Hannah Heil Funded by: EMBO - Postdoctoral Fellowships Duration: September 2021 - August 2023 Publications: 5 |
![]() |
Artificial Intelligence for Image Data Analysis in the Life Sciences Anna Kreshuk, Florian Jug, Ricardo Henriques, Wei Ouyang, Arrate Muñoz-Barrutia, Emma Lundberg, Matthew Hartley Alias: AI4Life Funded by: H2021 - INFRA Duration: September 2021 - August 2025 Publications: 19 |
![]() |
Optial Biology PhD programme Michael Hausser, Ricardo Henriques, Antonella Riccio Funded by: Wellcome Trust - 4-year PhD Programme in Science Duration: August 2021 - August 2025 |
![]() |
Mapping HIV-1 infection by 4D Super-Resolution Microscopy Hannah Heil Funded by: FCT - CEEC Individual Duration: July 2021 - June 2027 Publications: 3 |
![]() |
Unveiling live-cell viral replication at the nanoscale Ricardo Henriques Funded by: EMBO - Installation Grant Duration: January 2021 - January 2026 Publications: 27 |
![]() |
Developing AI for Microscopy Ricardo Henriques Funded by: NVIDIA - NVIDIA Academic Hardware Grant Program Duration: December 2018 - December 2018 |